TECH
What are the Challenges of Implementing Augmented Analytics?
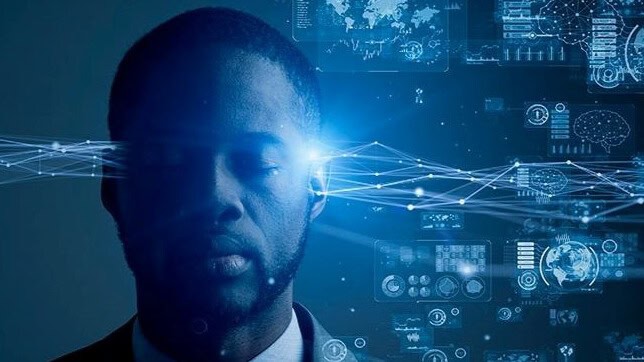
In the rapidly evolving data analytics landscapes, augmented analytics has developed as a revolutionary approach that uses artificial intelligence (AI) to enhance traditional data analysis methods. This innovative technology aims to automate data preparation, provide deeper insights, and simplify complex analysis processes. However, despite its potential to transform decision-making and streamline operations, implementing augmented analytics presents several challenges. This blog will explore these challenges in detail, providing insights into the obstacles organizations may face and offering strategies to overcome them. For those seeking expertise in this field, a Data Analytics Course in Chennai can provide valuable insights into these obstacles and offer strategies to overcome them. This blog will explore these challenges in detail, shedding light on the obstacles organizations may face and providing strategies for successful implementation.
Challenges of Implementing Augmented Analytics
Integration with Existing Systems
One of the primary challenges of implementing augmented analytics is integrating it with existing data systems and infrastructure. Many organizations have established data management processes and tools, and incorporating new technologies can be complex. Augmented analytics tools must seamlessly integrate with legacy systems, databases, and data warehouses to ensure a smooth transition and avoid disruptions. This often requires significant effort in terms of data migration, system compatibility, and customization.
Data Quality and Consistency
Augmented analytics relies heavily on data quality and consistency to generate accurate insights. Poor data quality, such as incomplete, outdated, or erroneous data, can undermine the effectiveness of augmented analytics. Organizations must invest in data cleaning and governance practices to ensure that the data fed into augmented analytics systems is accurate and reliable. Addressing data quality issues requires ongoing efforts and collaboration between data management teams and analytics professionals.
Skill Gap and Training
The implementation of augmented analytics often necessitates new skills and expertise. Data scientists, analysts, and IT professionals need to be proficient in using AI and ML algorithms, as well as understanding how to interpret and leverage the insights generated by these technologies. Organizations may face a skill gap, where existing staff need to gain the necessary knowledge to use augmented analytics tools effectively. Investing in training and developments programs is important to equip employees with the skills needed to harness the full potential of augmented analytics.
Cost and Resource Allocation
Implementing augmented analytics can be costly, particularly for organizations with limited budgets. The expenses associated with acquiring advanced analytics tools, integrating them into existing systems, and training personnel can be significant. Additionally, there may be ongoing costs relateds to maintenance, upgrades, and support. Organizations must carefully assess their budget and allocate resources efficiently to ensure a successful implementation without compromising other critical areas of their operations.
Change Management and Adoption
Introducing augmented analytics involves not only technological changes but also shifts in organizational culture and processes. Employees may refrain from adopting new technologies or altering their established workflows. Effective changes management strategies are essential to facilitate smooth transitions. This includes communicating the benefits of augmented analytics, addressing concerns, and providing support to help employees adapt to new tools and processes. Building a culture that embraces innovation and data-driven decision-making is key to overcoming resistance and achieving successful adoption.
Security and Privacy Concerns
Augmented analytics, like any technology that handles sensitive data, has security and privacy concerns. Protecting data from unwanted access, breaches, and misuse is vital. To protect data and meet standards, organizations must use strong security measures such as encryptions, access limits, and frequent audits. Ensuring data protection and building confidence with customers and stakeholders is critical to the effective adoption of augmented analytics.
Augmented analytics holds great promise for transforming data analysis and decision-making. However, its implementation comes with challenges, including system integration, data quality, skill gaps, cost management, change facilitation, and data security. A Data Analytics Course in Bangalore can provide essential skills and insights for those seeking to navigate these challenges effectively. By addressing these issues and developing effective strategies, businesses can utilize the full potential of augmented analytics. Embracing these challenges as growth opportunities will lead to successful implementation, driving valuable insights, innovation, and competitive advantage.
TECH
Understanding the 661 Area Code, Everything You Need to Know
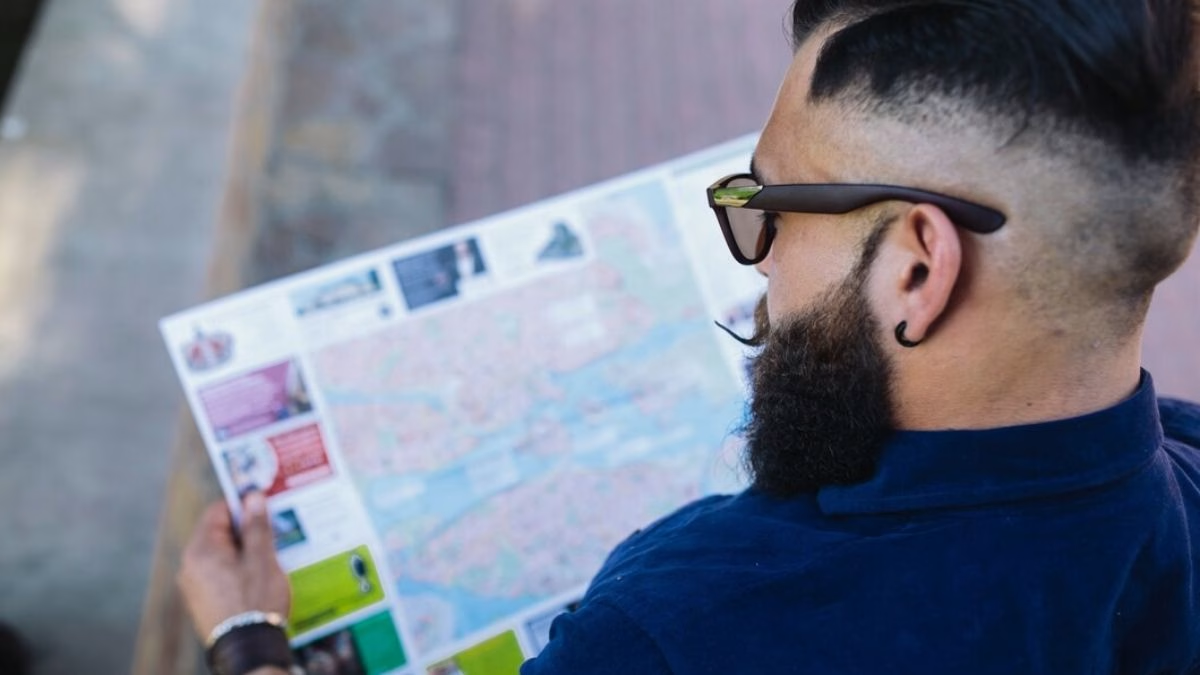
The 661 area code might seem like just another set of numbers, but it holds a unique significance in California. Whether you’re planning to move to an area within 661, trying to identify where a call came from, or ensuring you’re marketing to the right audience as a business owner, this guide will help you understand its importance.
This blog takes you through the origins of the code, its geographical span, some of the top cities it covers, and tips for navigating its connections. Let’s jump in!
What is the 661 Area Code?
The 661 area code is part of the North American Numbering Plan (NANP) and serves a diverse set of locations in central and southern California. It was created in 1999 when it split off from the 805 area code to accommodate the growing need for phone numbers in the region.
Think of the code as a connector—it serves residents, businesses, and visitors across a broad and diverse area, making it crucial for local communications.
The Geography of the 661 Area Code
Where is the 661 Area Code Located?
The 661 area code serves parts of Kern County and northern Los Angeles County. It also covers parts of San Bernardino County. While it might sound like a relatively small stretch, the area is geographically expansive.
Some of the most well-known cities and communities in the code include:
- Bakersfield: Known for its vibrant country music scene and agricultural market, Bakersfield is one of the largest cities covered by 661.
- Palmdale: A growing city, Palmdale is well-known for aerospace advancements and offers suburban living at its finest.
- Lancaster: Neighboring Palmdale, Lancaster boasts a community-focused atmosphere and stunning desert scenery.
- Tehachapi: Famous for its wind farms and scenic mountain views, Tehachapi is a small town but a recognizable stop within the area.
- Santa Clarita (parts): While most of Santa Clarita falls under the ode, this thriving city is a mix of innovation and suburban charm.
Rural Charm and Urban Growth
The 661 area code offers a fascinating blend of rural communities and urban centers. It’s notable for its agricultural roots, particularly in Bakersfield and the surrounding Kern County, but it has also become a hub for suburban families and businesses in the Antelope Valley cities like Palmdale and Lancaster.
Why Does the 661 Area Code Matter?
For Residents
If you live or plan to move within a code city, this number will likely become a part of your identity. Locals often take pride in their area codes, which can reflect the unique vibe of the region they call home.
For Businesses
For businesses operating in the 661 area code, having a local phone number is essential for creating trust and familiarity with customers. Whether it’s a startup in Palmdale or an agricultural supplier in Bakersfield, the 661 area code can help establish a solid local presence.
For Travelers
If you’re visiting for work or leisure, understanding the area code could save you from confusion when dialing local numbers. You’ll also know exactly where a call or text is coming from if you see 661 on your screen.
Fun Facts About the 661 Area Code
- Cultural Significance: Bakersfield, the largest metropolitan area within 661, is the birthplace of the “Bakersfield Sound,” a sub-genre of country music made famous by Merle Haggard and Buck Owens.
- Nature Meets Progress: The area includes both natural wonders, such as the Mojave Desert and Tehachapi Mountains, and industrial innovation through aerospace developments in areas like Mojave.
- Hollywood’s Backyard: Parts of the Antelope Valley in 661 have served as filming locations for countless movies and TV shows due to their stunning desert landscapes.
Navigating the 661 Area Code
How to Get a 661 Phone Number
If you’re moving or starting a business in the region, it’s easy to set up a local phone number:
- Contact Your Carrier: Notify your mobile carrier or landline provider about setting up a line in the 661 area.
- VoIP Options: Services like Google Voice and providers of Voice over Internet Protocol (VoIP) can assign you a 661 number even if you aren’t physically in the area.
- Keep It Local: If you’re running a business, having a local area code instills confidence and encourages engagement from nearby customers.
Recognize Potential Spam Calls
Like many area codes, 661 isn’t immune to spam or robocalls. Always verify unknown numbers before answering and use call-filtering apps to minimize disruptions.
The Future of the 661 Area Code
With growing populations and increased demand for phone numbers, area codes need to evolve. While the 661 has served its community well for over two decades, California’s continued development may eventually require new splits or overlays in the future. This ensures businesses and residents alike can stay connected without interruptions.
Discover the Beauty of the 661 Area Code
The 661 area code is much more than just a number. It’s a symbol of a region filled with rich history, growing opportunities, and a strong sense of community. Whether you’re dialing in from the busy streets of Bakersfield or the peaceful mountain ranges of Tehachapi, 661 connects people and places seamlessly.
Are you curious about areas or area codes near 661? Drop us a comment, or explore our [Ultimate Guide to California Area Codes] for more insights!
TECH
Everything You Need to Know About the 954 Area Code
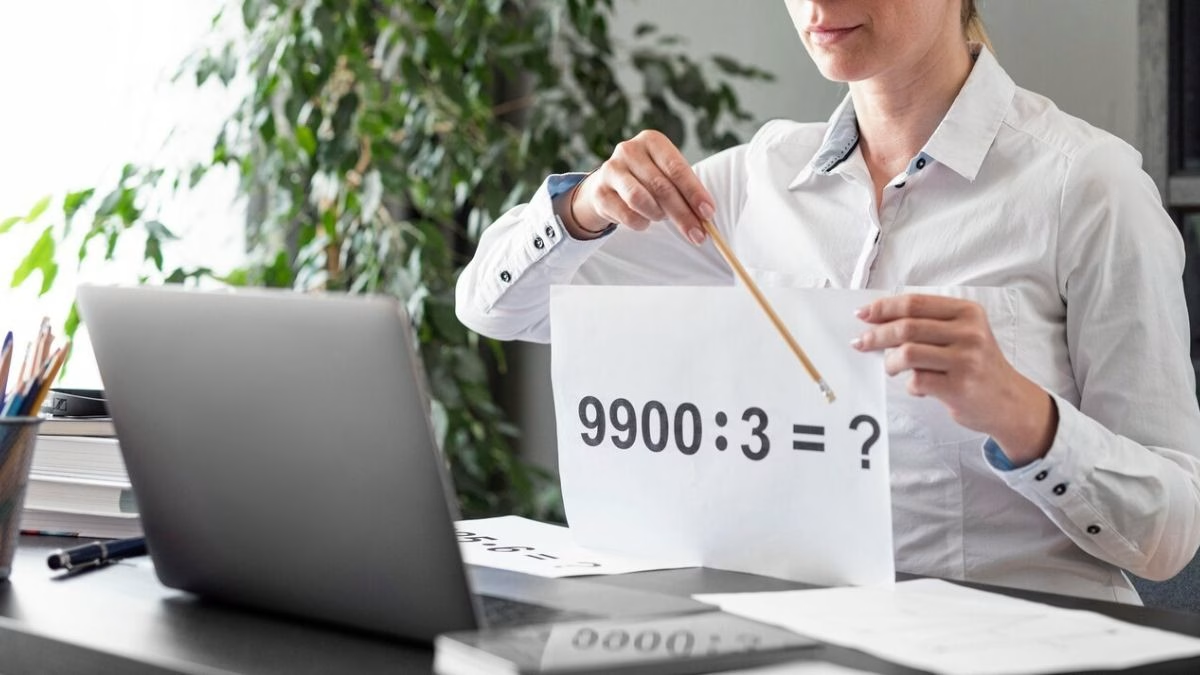
Are you dialing or receiving calls from a 954 number? Whether you’re curious about its history, the regions it covers, or how it fits into your daily communications, we’ve got all the answers you need right here! This guide will provide you with an easy-to-understand overview of the 954 area code, its significance, and its practical applications.
By the end of this post, you’ll know everything you need to make the most of this widely-used area code.
What Is the 954 Area Code?
The 954 area code is a telephone area code in Florida, part of the North American Numbering Plan (NANP). It primarily covers Broward County, including major cities like Fort Lauderdale, Hollywood, Pompano Beach, and Coral Springs. Introduced in 1995, the code was separated from the 305 area code to accommodate the growing population and demand for phone numbers in South Florida.
Over time, due to the continued surge in population and telecommunication needs, the code was met with such high use that an additional overlay area code (754) was introduced in 2002 to ensure a steady supply of phone numbers for the region.
Which Cities and Areas Use the 954 Area Code?
The 954 area code proudly serves Broward County, Florida. Here are some of the key cities included within its range:
- Fort Lauderdale
Known as Florida’s “Venice of America” for its world-class boating canals, Fort Lauderdale boasts stunning beaches and a vibrant cultural scene.
- Hollywood
This charming city offers a unique mix of laid-back beachfront vibes and urban sophistication, paired with its famous Broadwalk.
- Coral Springs
Nestled to the northwest of Broward County, Coral Springs is a family-friendly community with nature parks and top-rated schools.
- Pompano Beach
A haven for water sports and fishing enthusiasts, Pompano Beach represents a historical nexus for South Florida tourism.
Beyond these, the 954 area code extends its communication coverage to various other suburbs and vibrant neighborhoods within Broward County.
Why Was the 954 Area Code Created?
With technological progress and population growth, the original 305 area code—which originally covered all of South Florida—became overstretched. By 1995, Florida’s development had skyrocketed, driven by its beaches, tourism, and influx of residents. To manage this growth, the 305 area code was divided, assigning Broward County its own unique code—954.
The separation ensured robust service coverage and an organized numbering plan, further ensuring that South Florida would remain one of the most efficiently connected regions.
Interesting Facts About the 954 Area Code
- Overlay with 754: The 954 area code is shared or “overlaid” with the 754 area code, allowing the same geographical area to support more phone numbers. If you see a 754 dialed or received alongside 954, they originate from the same region.
- Cultural Hub: Broward County (and, by extension, the 954 area code) is considered a cultural hotspot in Florida. It’s home to some of the state’s major festivals, museums, and lifestyle events, making it iconic for more than just its area code.
- Business and Tourism: Aside from being home to hundreds of thousands of residents, the code ambitiously supports one of Florida’s largest economies—tourism. It’s heavily tied to businesses in hospitality, ranging from luxurious waterfront hotels to vibrant event spaces.
Common Uses of the 954 Area Code
Residential Use
Whether you’re making a call within your neighborhood or connecting across South Florida, the code is standard for those living in Broward County. It provides a reliable connection that ensures households can access modern telecommunication services.
Business Use
Local businesses thrive under the code, tapping into the connectivity it offers to communicate with clients, manage operations, and foster relationships. Marketing agencies, tech startups, e-commerce hubs, and medical centers frequently use 954 numbers to build trust among their local clientele.
Mobile Numbers
Smartphone users in Broward County—spanning generations—commonly assign the code to their personal and work numbers. It ensures quick identification and accepts a wide range of services, spanning calls, text, and apps.
How to Dial Within and To the 954 Area Code
If you’re dialing:
Locally Within 954
When calling someone in the same area code (including the 754 overlay), dial the 10-digit number directly (e.g., 954-XXX-XXXX). No need to add “1” before the number unless your phone system requires it.
From Outside the 954 Area Code
If calling from another area code—or another state—dial 1 + 954 + the seven-digit number. For international callers, include “+1” before the area code when dialing (e.g., +1-954-XXX-XXXX).
Managing Calls From the 954 Area Code
If you receive a call from a 954 number and aren’t sure whether it’s a legitimate contact, consider the following quick steps:
- Check Caller ID First. Verify the number against your contacts list to ensure familiarity.
- Be Aware of Scams. Some scam callers “spoof” area codes to appear local. If something feels off, don’t engage—simply hang up and report.
- Call Back If Needed. Use discretion when returning calls to unknown 954 numbers. Confirm the caller’s identity before sharing personal details.
How to Get a 954 Number
Thinking of acquiring a 954 number for yourself or your business? It’s a straightforward process if you’re based in Broward County. Here’s how you can get started:
- Contact a Service Provider: Major telecom providers like AT&T, Verizon, and T-Mobile can set up a 954 number during your initial sign-up.
- Switch Your Current Number: If you’re moving to Broward County, your provider can port an existing number into a newly allocated 954 number.
- Check for Availability: Ensure that the 954 area code is available. If it’s not, consider the equally local-friendly overlay code, 754.
Enhancing Your Connection to South Florida
Whether you’re a current resident, a business owner, or just curious, the 954 area code is much more than digits—it’s the communication backbone of a thriving, vibrant Florida community. By understanding its history, key uses, and dialing basics, you’re a step closer to connecting meaningfully with the people and culture behind this iconic code.
Want to learn more about area codes, phone plans, or regional telecom? Browse our additional resources and unlock a world of information to keep you informed and connected.
TECH
Honor Magic 5 Pro Review by Geekzilla.tech
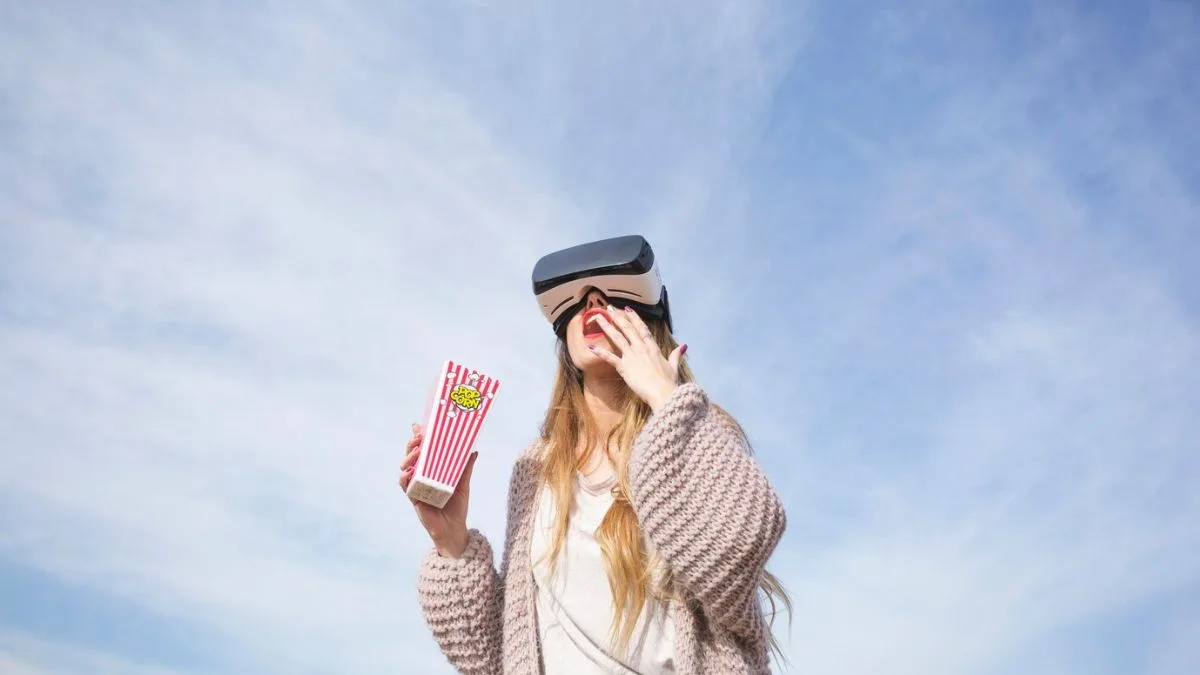
Honor has made a name for itself with sleek designs and cutting-edge specifications, and the Honor Magic 5 Pro is no exception. For tech enthusiasts at heart, Geekzilla.tech dives deep into the Magic 5 Pro, uncovering how it stands out in the competitive flagship smartphone market of 2024.
What Makes the Honor Magic 5 Pro Stand Out?
The Honor Magic 5 Pro is designed to impress, boasting advanced features for those who value top-tier performance and functionality. Whether you’re into mobile photography, gaming, or simply need a reliable everyday device, this smartphone aims to check all the boxes.
Key Features of the Honor Magic 5 Pro:
- Powerful Processor: Packed with one of the latest chipsets, the Magic 5 Pro by Geekzilla.tech ensures smooth multitasking, lag-free gaming, and seamless performance for demanding apps.
- Impressive Camera System: Known for its high-quality cameras, the Magic 5 Pro offers exceptional photography capabilities, making it ideal for creating stunning visuals.
- Long-Lasting Battery: A powerhouse that can easily last through a full day of heavy usage without constant recharging.
- Innovative Display Technology: Boasts a vivid, high-resolution display with a smooth refresh rate, perfect for immersive entertainment and crystal-clear visuals.
Seen by many tech enthusiasts as a versatile flagship, the Honor Magic 5 Pro has generated substantial curiosity amongst users eager to have comprehensive performance in their pockets.
A Closer Look at What Matters
1. Performance: Powered for Anything
The Magic 5 Pro is equipped with a high-performance processor, ensuring fluid operation across apps, games, and multitasking. This, combined with its efficient cooling system, minimizes lag and keeps your device performing at its peak all day.
2. Display Excellence
The edge-to-edge display promises vibrant colors, deep contrasts, and a high refresh rate. Whether you’re watching videos, playing games, or scrolling through social media, this phone’s screen is designed to provide an optimal viewing experience.
3. Camera Features That Deliver
With its advanced triple-camera setup, the Magic 5 Pro excels in capturing high-quality photos in both bright and low-light conditions. Features such as AI-assisted photography ensure that every shot is flawless with minimal effort.
4. Battery and Charging
A flagship is nothing without a reliable battery. The Honor Magic 5 Pro boasts an all-day battery life with fast-charging technology, meaning less time plugged in and more time enjoying what you love doing.
5. Design That Turns Heads
Honor has always focused on offering stylish yet functional designs. The Magic 5 Pro follows suit with a slim, ergonomic design that feels premium in your hand. Paired with a range of striking color options, this phone is as much a style statement as it is a tech powerhouse.
Is the Honor Magic 5 Pro Worth It?
For individuals searching for a device that balances performance, design, and functionality, the Honor Magic 5 Pro is undeniably a strong contender. It stands out as a premium choice for users who refuse to compromise on quality or aesthetics.
Whether you’re a tech enthusiast, a content creator, or someone who simply wants a reliable flagship phone, the Honor Magic 5 Pro caters to a wide range of needs while offering excellent value for its price.
Final Thoughts
The champions innovation, making it an attractive option for users seeking premium features in their next smartphone. With its cutting-edge camera system, performance-centric hardware, and sleek design, it raises the bar in the flagship smartphone arena.
Looking to enjoy ultimate performance in your next phone? Based on its specs, Geekzilla.tech enthusiastically endorses the Honor Magic 5 Pro as a device worth considering.
-
GAME5 months ago
Mastering the Fire Kirin Login Experience
-
BLOG7 months ago
Pepper0 Mother and Son
-
BUSINESS7 months ago
Why Your Business Needs an Elite Eagle Product Partner for Growth
-
BLOG9 months ago
Czech Harem: Understanding the Controversial Phenomenon
-
FASHION9 months ago
old fashioned news source nyt crossword
-
BLOG10 months ago
Bunkr Albums: A Comprehensive Guide
-
NEWS9 months ago
spencer new york news: A Comprehensive Guide
-
BLOG6 months ago
EntreTech.org: Empowering Entrepreneurs Through Technology